Exploring Mobile App Updates: An International Study on User Behavior and Adoption
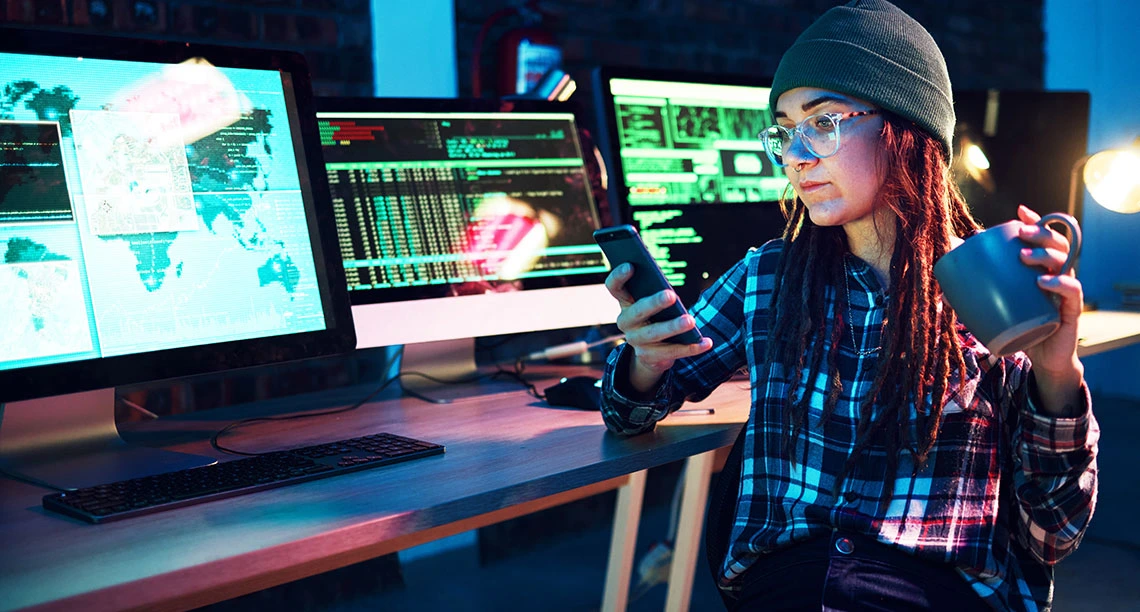
In the ever-evolving landscape of mobile technology, understanding how users adopt new app versions is crucial for developers. Xuan Lu, an assistant professor at the University of Arizona College of Information Science, has contributed significantly to this field through a collaborative, international study on the adoption of “recurrent innovations”—technological updates that occur frequently and successively, often at short intervals—focusing on mobile app updates. This comprehensive research provides novel insights into user behavior, challenging existing theories on “innovation diffusion,” or the process by which a new technology spreads through a population of users.
A New Perspective on Innovation Adoption
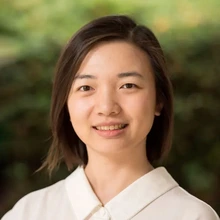
Xuan Lu, Assistant Professor in the University of Arizona College of Information Science.
The study, published in the peer-reviewed journal ACM Transactions on the Web, is titled “Adoption of Recurrent Innovations: A Large-Scale Case Study on Mobile App Updates.” It explores the unique characteristics of recurrent innovations. Unlike traditional single or successive innovations, recurrent innovations—such as mobile apps—are characterized by their rapid and continuous development cycle, often receiving updates every few weeks or even days. These updates can include new features, bug fixes and user interface improvements, and users can easily adopt the latest version with minimal effort, typically just a click on their smart devices. This frequent updating cycle differentiates recurrent innovations from single or successive innovations, which often have more extended periods between new iterations. The research team, led by Lu and comprising scholars from Peking University, the University of Maryland and the University of Michigan, utilized a vast dataset of millions of Android users to analyze their adoption patterns across a wide range of apps.
Key Findings: New Categories of Adopters
The study reveals that existing models of innovation diffusion, such as Everett Rogers’ widely referenced Diffusion of Innovations theory, do not fully explain the adoption patterns of recurrent innovations. Rogers’ model categorizes adopters into innovators, early adopters, early majority, late majority and laggards. However, Lu and her colleagues identified three new categories specific to recurrent innovations:
- Immediate-Adopters: These users update to new versions almost instantly, often within a day. They may have auto-update settings enabled or be highly engaged with the app.
- Preservers: These users continue to use an older version despite the availability of newer ones. Their behavior may stem from satisfaction with the current version or reluctance to change.
- Retro-Adopters: These users revert to an earlier version after initially adopting a newer one, indicating dissatisfaction with the updates.
These new adopter categories highlight the complex dynamics in user interactions with frequently updated technologies.
Certain factors significantly influence whether and when users adopt new versions. Key predictors include the frequency of past updates, user engagement levels and the app’s release history.
Predictive Analysis and Practical Implications
To further understand adoption behavior, the research team developed predictive models using machine learning. They found that certain factors significantly influence whether and when users adopt new versions. Key predictors include the frequency of past updates, user engagement levels and the app’s release history.
For app developers, these findings offer practical strategies:
- Tailored Update Strategies: Understanding user behavior can help developers decide when to release updates and how to encourage adoption.
- User Engagement: Enhancing user engagement can lead to quicker adoption of new versions.
- Targeted Promotions: Predictive models can help identify users who are likely to update, allowing for targeted marketing efforts.
Conclusion
The team’s research sheds light on the intricate patterns of mobile app update adoption, extending existing theories and offering new frameworks for understanding user behavior. By identifying immediate-adopters, preservers and retro-adopters, the study provides valuable insights on recurrent innovations for both academic researchers and industry practitioners.
This work not only advances the theoretical understanding of innovation diffusion but also equips developers with tools to optimize app update strategies, ultimately enhancing user experience and engagement.