The Intricacies of Human Language: 5 Questions with Associate Professor Steven Bethard
INFOSCI FACULTY PROFILE
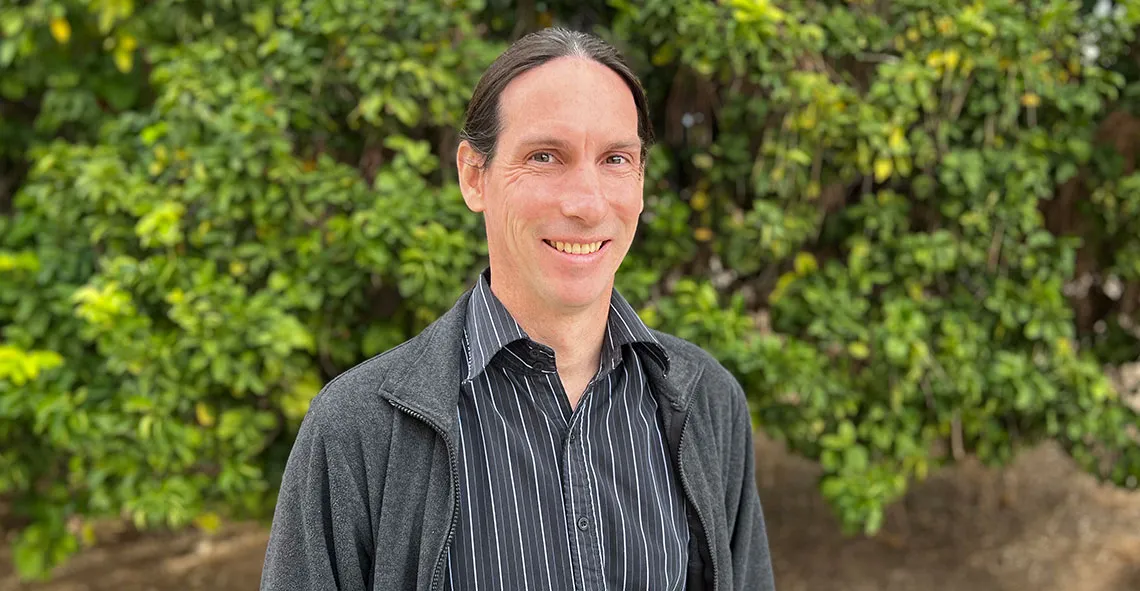
Steven Bethard, Associate Professor.
What is exciting about my research is the challenge of teaching machines the intricacies of human language: not just the ability to generate fluent language as large language models do, but to actually understand language and be able to formalize that understanding into a computer-friendly structured representation.
Associate Professor Steven Bethard joined the College of Information Science in 2016 after serving as an assistant professor of computer and information science at the University of Alabama at Birmingham and a postdoctoral researcher at Stanford University’s Natural Language Processing group, as well as Johns Hopkins University, KULeuven in Belgium and the University of Colorado. Dr. Bethard’s research focuses on natural language processing and machine learning theory and applications, and at the College of Information Science he teaches Neural Networks, advises students in the PhD in Information and oversees bachelor’s and master’s student directed research.
What is your current research, and what most excites you about this work?
My research interests include natural language processing and machine learning theory and applications, including modeling the language of time and timelines, normalizing text to medical and geospatial ontologies, and information extraction models for clinical applications.
What is exciting about this work is the challenge of teaching machines the intricacies of human language: not just the ability to generate fluent language as large language models do, but to actually understand language and be able to formalize that understanding into a computer-friendly structured representation.
Tell us about a research project that you find particularly rewarding.
Temporal relation discovery for clinical text is a National Institutes of Health award that I have been a part of since it started in 2010. Since its inception, the project has pushed the boundaries of temporal relation extraction from the Electronic Medical Records clinical narrative by investigating new computational methods within the context of the latest developments in the fields of natural language processing, machine learning, artificial intelligence and biomedical informatics. Designing machine learning models for extracting timelines from clinical notes is still one of the core elements of my research.
What are you teaching, and what do you most enjoy about teaching?
Though I’m not teaching this semester, last semester (and most years) I teach Neural Networks. I love teaching this class because it’s a hands-on exploration of exactly how deep learning models work and the students are always so engaged. The diversity of learners (from astronomers to linguists to information scientists) means there are always new, smart questions to dig deeper into.
How do you bring your research into your teaching?
My research relies heavily on neural networks, so many of the examples in the Neural Networks course are drawn from my own research area, natural language processing. The graduate students in that course participate in a class-wide competition, whose data is often drawn from one of my own research projects.
How do you engage with students to foster their academic and professional growth in and out of the classroom?
Inside the classroom, my courses always include hands-on activities, where students get to try out techniques with guidance from myself and the teaching assistant. Outside of the classroom, I regularly take on undergraduate, master’s and doctoral students for one-semester directed research and capstone projects.
Learn more about Steven Bethard on his faculty page or GitHub site, or explore ways you can support the dynamic faculty of the College of Information Science and their research and teaching.