How Well Does AI Conduct Research? Meet the Researcher Trying to Find Out
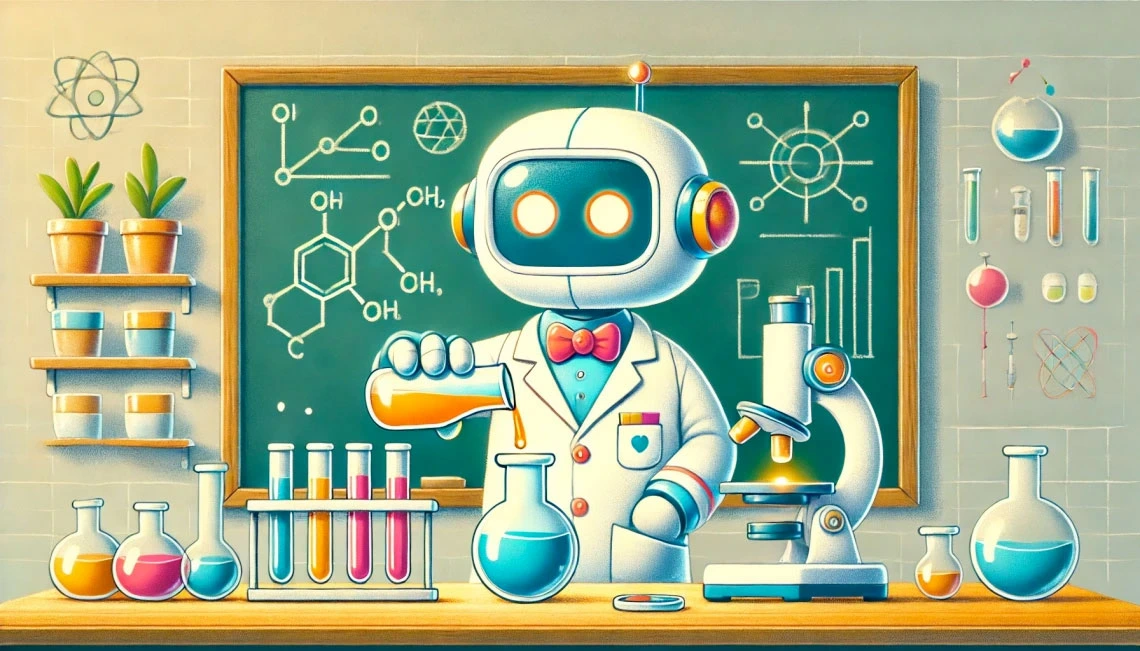
For now, the answer is clear: AI cannot yet match humans in research. But the challenge is more nuanced, says Peter Jansen, an associate professor in the College of Information Science, who is finding ways to measure how effectively computers create new knowledge.
This image was generated by DALL-E using ChatGPT.
About a decade ago, Peter Jansen and others who study artificial intelligence tested early AI models to see if they could solve grade-school multiple choice exams from 12 U.S. states.
Over years, hundreds of AI researchers, including Jansen, worked to improve the performance of AI models on the exam questions, eventually reaching 80%-90% accuracy. But when asked to show their work, the models struggled. It took years of experiments for the models to provide correct explanations for their answers to fourth grade science questions just 30% of the time.
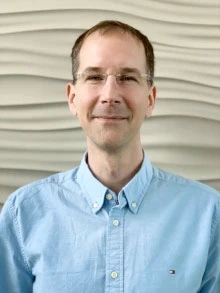
Peter Jansen, Associate Professor, College of Information Science, The University of Arizona.
The idea of AI performing scientific reasoning as well as humans seemed far off. Then, two years ago, OpenAI's ChatGPT arrived, drastically changing the landscape. AI's performance on the science exam benchmark skyrocketed from 30% to 97% almost overnight.
"For all of us who had been working on this for a decade or more, overnight, the performance basically solved whatever tasks we were working on," says Jansen, an associate professor in the College of Information Science. "So, a bunch of us really needed to find new things to do."
Jansen's new focus sits squarely at the intersection of AI and research, testing how well AI performs at scientific discovery compared with humans. His goal is to measure how effectively computers create new knowledge.
For now, the answer is clear: AI cannot yet match humans in research. But the challenge is more nuanced, Jansen says: "We don't know what we don't know. Nobody has a good way of doing research in this field right now because we don't have the research methods to be able to actually know when a thing works or not."
Checking the Accuracy of New Knowledge
Evaluating AI's capabilities can be straightforward for everyday tasks. For example, an AI tool diagnosing a disease can be double-checked by a doctor. But science operates at the edge of human knowledge, making evaluation much harder, says Jansen, who holds an appointment with the Allen Institute for Artificial Intelligence, known as Ai2, a nonprofit research institute in Seattle.
One method of evaluation Jansen developed is DiscoveryWorld, a virtual environment that looks like a game where AI models such as ChatGPT pretend to be scientists and perform simulated scientific discovery tasks. Set on the fictional Planet X, the program asks AI to tackle challenges ranging from translating alien languages to diagnosing why local space food is making researchers sick.
In a recent study conducted with DiscoveryWorld, Jansen's team compared AI's performance on these tasks with that of human researchers with advanced degrees. Human scientists successfully completed the moderately difficult and most difficult tasks about 60%-70% of the time; the humans were not asked to complete the easiest tasks, which were extremely rudimentary. The AI models, on the other hand, completed the moderate and most difficult tasks between just 10%-20% of the time. The best AI score, on one of the easiest tasks, was about 40%.
But much like when he was testing AI models using grade-school science exams, Jansen says seeing the AI models' work is more valuable than the answers. DiscoveryWorld keeps track of everything the AI agent does, allowing researchers to see what aspects of science they're good at, and what aspects they still fall short in.
"They're kind of bumbling around. They're doing things generally that they ought to be doing, but they're not necessarily putting the big picture together," he says, which is a much more useful takeaway than "the AI didn't work."
"But if you have finer grained measures, like we do here, you can say, 'Oh yeah, it's really good at using instruments, but it's really bad at collecting samples,'" Jansen adds. "As a scientist, I can work with that."
Stepping Stones Toward Improvement
With human-conducted research, evaluation comes during the peer-review process. It can't work that way with AI-conducted research, Jansen says.
"They assume that you, as a human being who's got a PhD and a bunch of training, know what you're doing, and that you're not going to make stupid mistakes," he says, referring to publications' peer reviewers. "With an AI model, that's not the case at all. It could be really good at things that take human expertise to be good at, but it can be really bad at a lot of the stepping stones along the way."
Relying on AI for research now, Jansen says, is akin to asking a random person off the street to assist with a study, Jansen said.
Yet he remains optimistic.
"These things could be amazing, and I have every confidence that in the near future—I don't know if that's in a year, in five years, in 10 years—they're going to be making discoveries," he says. "But before they do, we need to be able to measure how good they are."
Learn more about the cutting-edge research of College of Information Science faculty, or explore ways you can support faculty and student research.