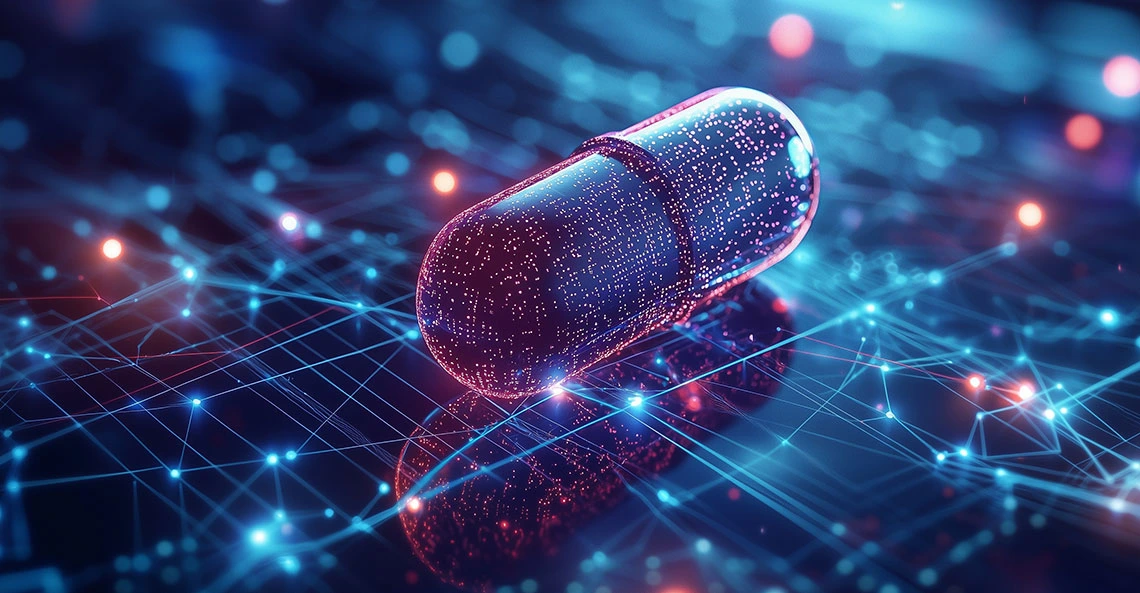
When
Where
Join us in Harvill 460 for the College of Information Science Colloquium Series, featuring Travis Wheeler, University of Arizona Associate Professor of Pharmacy Practice and Science and Director of the Wheeler Lab.
Deep learning techniques have produced remarkable breakthroughs across a diverse space of challenging problems.* Based on such successes, it is natural to expect that deep learning methods will similarly lead to dramatic advances in the accuracy of methods for rapidly predicting the interaction potential of a target protein and a drug candidate. In this talk, Dr. Travis Wheeler will discuss some advances along these lines, and work that he and his team are performing to gather massive new data sets to train the next generation of deep learning methods for virtual screening.
* Note: Wheeler asked an AI chat tool to create a haiku describing my research. It gave me the following ... then provided the second as a bonus:
Protein worlds unfold,
AI guides through vast landscapes,
Cures within our grasp.
Simulations dance,
Molecules in vibrant waltz,
Discovery awaits.
About Travis Wheeler
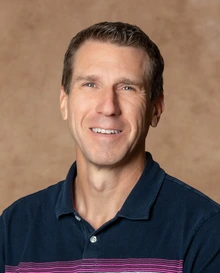
Dr. Travis Wheeler has over 25 years of experience in research in designing algorithms, statistical models and software for problems motivated by biological data. Projects in his research group range from statistical modeling of biological sequence families, to text indexing and bounded search algorithms, to low-level software optimization, to Deep Neural Networks and Natural Language Processing and related Machine Learning methods, to development of databases and accompanying web services. These efforts are aimed at improving insight into genomic and proteomic data, computational drug discovery methods, analysis of mass spectrometry data and automated animal tracking and behavior classification.
Header image courtesy Adobe Stock.