Research Led by Information PhD Student Ruoyao Wang Uncovers Limitations in Using Language Models as World Simulators
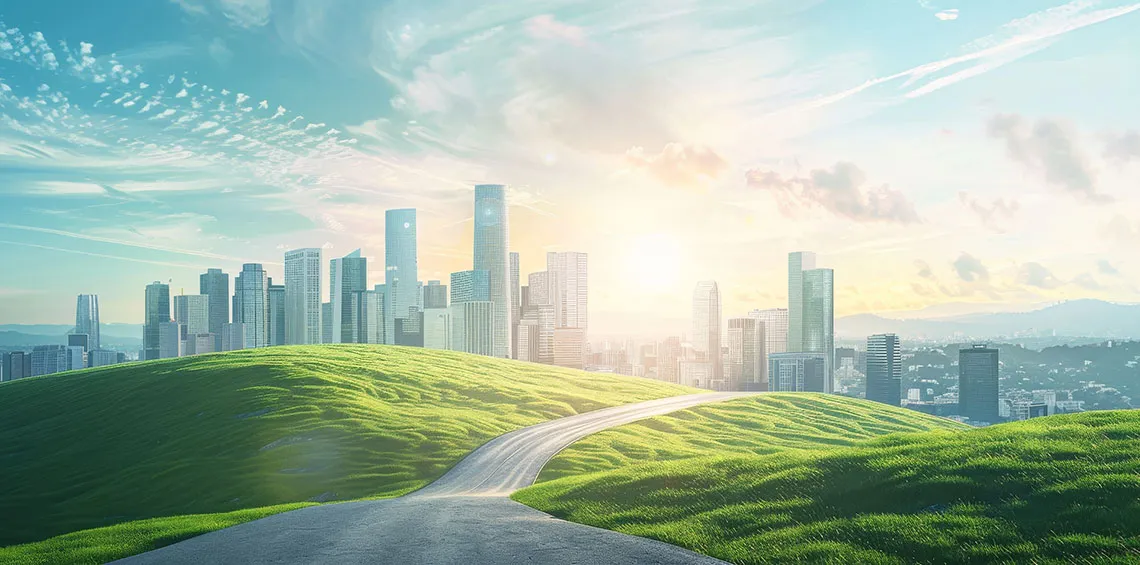
Image by apirom, generated with AI, courtesy Adobe Stock.
In the ever-evolving world of artificial intelligence, researchers constantly seek new ways to make machines understand and simulate our reality. Among those at the forefront is Ruoyao Wang, a fourth-year PhD in Information student in the University of Arizona’s College of Information Science. Wang’s recent study, alongside colleagues from New York University, Johns Hopkins University, Microsoft Research and the Allen Institute for AI, sheds light on a critical question: Can large language models (LLMs), such as OpenAI’s GPT-4, act as reliable world simulators?
The short answer: not yet.
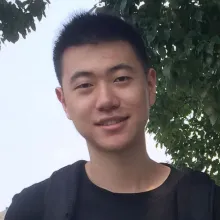
Ruoyao Wang, PhD in Information, The University of Arizona.
Their findings, presented at the 62nd Annual Meeting of the Association for Computational Linguistics and recently highlighted in the State of AI Report 2024, reveal that even the most advanced LLMs struggle with accurately simulating real-world dynamics, especially when tasked with complex planning or state transitions.
World simulation, in this context, refers to the ability of an AI system to predict how actions—whether as simple as turning on a faucet or as complex as navigating a virtual environment—alter the state of a given world. Wang and his collaborators, which includes University of Arizona Associate Professor Peter Jansen, tested these abilities through text-based virtual environments, a digital echo of old-school computer games. Their goal was to see whether LLMs could correctly predict the results of various actions, bypassing the need for complex manual coding.
The team’s research specifically centers on "text virtual environments," which use natural language to simulate real-world phenomena. These environments offer a crucial testing ground for AI models' ability to understand and predict real-world behavior. However, the study revealed that while GPT-4 could predict direct action outcomes (like whether turning on a sink would cause water to flow) with a success rate of 77%, it struggled with more complex environmental consequences, such as whether the water would fill a cup under the faucet. In these cases, the model’s accuracy dropped to just 50%.
A deeper look at the research reveals why these tasks are so challenging for LLMs. Wang and his collaborators introduced a novel benchmark, "BYTE-SIZED32-State-Prediction," which includes over 75,000 state transitions across 31 text-based games. These games, designed to simulate scientific and common-sense reasoning, tested the AI's ability to predict not only how an action (such as turning on a sink) changes the immediate state but also secondary, environment-driven consequences like a cup filling with water. While GPT-4 performed reasonably well on action-driven changes, its accuracy plummeted on environment-driven transitions—particularly those requiring common sense, physics or logical inference.
The research’s broader implications are significant. Wang and his team’s work highlights a key challenge: error accumulation. Even models with an initial accuracy rate of around 60% quickly degrade in performance after several sequential steps. For example, in simulations requiring 10 state transitions, GPT-4’s overall accuracy dropped below 1%—a major obstacle for AI systems aiming to simulate realistic environments over time. This finding underscores the limitations of current LLMs when it comes to reliably modeling complex, multi-step processes in dynamic environments.
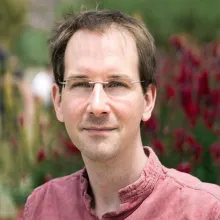
Peter Jansen, Associate Professor, College of Information Science, The University of Arizona.
Jansen, Wang’s PhD advisor, emphasizes the importance of the research led by Wang in the broader AI landscape. "Through the last three years in the PhD program at the College of Information Science, Ruoyao Wang has been able to develop unique expertise in using language models to simulate the world, as well as using language models as agents for performing tasks in virtual environments,” he says. “That expertise has become in extreme demand in the last six months, as the latest versions of (for example) GPT-4 have demonstrated the ability to work with virtual tools to perform tasks like code generation, autonomous web browsing or other tasks that normally require humans to perform."
Wang’s path to becoming a leading doctoral student in AI research reflects the dedication and focus he brings to his work. Before pursuing his PhD in Information at the University of Arizona, Wang earned an MS in Computer Science from the University of Michigan and a BS in Microelectronic Science and Engineering from Fudan University in Shanghai. His academic journey has been defined by a deep interest in natural language processing (NLP), particularly the intersection of AI and virtual environments. Prior to his work in NLP, Wang also gained research experience in medical image processing and embedded systems—diverse areas that underscore his multidisciplinary approach to AI challenges. His current focus, using AI to build simulations of the real world for scientific discovery, highlights the broad implications of his work.
Wang aims to create AI tools that can assist in everything from training environments to the study of human behaviors, positioning him at the cutting edge of AI innovation. “The goal of my research is to build up a simulation for the real world that we can use for scientific discovery,” he says.
While Wang’s research shows that LLMs like GPT-4 are not yet ready to simulate complex environments consistently, the findings are a crucial stepping stone. The team’s work opens the door to future innovations that could eventually make these models reliable world simulators, which could play an important role in fields ranging from basic and applied science to the development of autonomous systems.
Wang’s collaborators on this groundbreaking study include Jansen, who is also a member of the Allen Institute for AI, plus Graham Todd from New York University, Ziang Xiao from Johns Hopkins University, Xingdi Yuan and Marc-Alexandre Côté from Microsoft Research Montréal and Peter Clark from the Allen Institute for AI. Together, they form a multi-institutional research team pushing the boundaries of what AI systems can achieve.
While there are clear limitations in current large language models, the research led by Wang marks an important step toward understanding LLMs’ potential as world simulators. The team's findings highlight both the promise and the challenges of using AI to model complex environments, underscoring the need for further innovations to make these systems more reliable. As researchers continue to refine these models, this work serves as a foundation for future breakthroughs in AI-driven simulations, with wide-ranging applications from virtual training environments to scientific exploration. By identifying the gaps in current models, Wang and his colleagues are contributing valuable insights that will shape the next generation of AI capabilities.
Learn more about the University of Arizona PhD in Information, or explore the College of Information Science’s interdisciplinary research by leading faculty and researchers.